Invited speakers
Dr Li Deng, Principal Researcher, Microsoft Research, USA
Dr Niko Brümmer, Chief Scientist, AGNITIO, South Africa
Plenary Session 1

Dr Li Deng
Principal Researcher
Microsoft Research, USA
Being Deep and Being Dynamic --- New-Generation Models and Methodology for Advancing Speech Technology
An APSIPA Distinguished Lecture 2012
Semantic information embedded in the speech signal --- not only the phonetic/linguistic content but also a full range of paralinguistic information including speaker characteristics --- manifests itself in a dynamic process rooted in the deep linguistic hierarchy as an intrinsic part of the human cognitive system. Modeling both the dynamic process and the deep structure for advancing speech technology has been an active pursuit for over more than 20 years, but it is not until recently (since only a few years ago) that noticeable breakthrough has been achieved by the new methodology commonly referred to as "deep learning". Deep Belief Net (DBN) is recently being used to replace the Gaussian Mixture Model (GMM) component in HMM-based speech recognition, and has produced dramatic error rate reduction in both phone recognition and large vocabulary speech recognition while keeping the HMM component intact. On the other hand, the (constrained) Dynamic Bayesian Net (referred to as DBN* here) has been developed for many years to improve the dynamic models of speech while overcoming the IID assumption as a key weakness of the HMM, with a set of techniques and representations commonly known as hidden dynamic/trajectory models or articulatory-like models. A history of these two largely separate lines of "DBN/DBN*" research will be critically reviewed and analyzed in the context of modeling deep and dynamic linguistic hierarchy for advancing speech (as well as speaker) recognition technology. Future directions will be discussed for this exciting area of research that holds promise to build a foundation for the next-generation speech technology with human-like cognitive ability.
Short Biography
Li Deng received the Ph.D. from Univ. Wisconsin-Madison. He was an Assistant (1989-1992), Associate (1992-1996), and Full Professor (1996-1999) at the University of Waterloo, Ontario, Canada. He then joined Microsoft Research, Redmond, where he is currently a Principal Researcher and where he received Microsoft Research Technology Transfer, Goldstar, and Achievement Awards. Prior to MSR, he also worked or taught at Massachusetts Institute of Technology, ATR Interpreting Telecom. Research Lab. (Kyoto, Japan), and HKUST. He has published over 300 refereed papers in leading journals/conferences and 3 books covering broad areas of human language technology, machine learning, and audio, speech, and signal processing. He is a Fellow of the Acoustical Society of America, a Fellow of the IEEE, and a Fellow of the International Speech Communication Association. He is an inventor or co-inventor of over 50 granted US, Japanese, or international patents. He served on the Board of Governors of the IEEE Sig. Proc. Soc. (2008-2010). More recently, he served as Editor-in-Chief for IEEE Signal Processing Magazine (2009-2011), which, according to the Thompson Reuters Journal Citation Report released 2010 and 2011, ranked first in both years among all 127 IEEE publications and all 247 publications within the Electrical and Electronics Engineering Category worldwide in terms of its impact factor, and for which he received the 2011 IEEE SPS Meritorious Service Award. He currently serves as Editor-in-Chief for IEEE Transactions on Audio, Speech and Language Processing. His recent tutorials on deep learning at APSIPA (Oct 2011) and at ICASSP (March 2012) received the highest attendance rate at both conferences.
Plenary Session 2
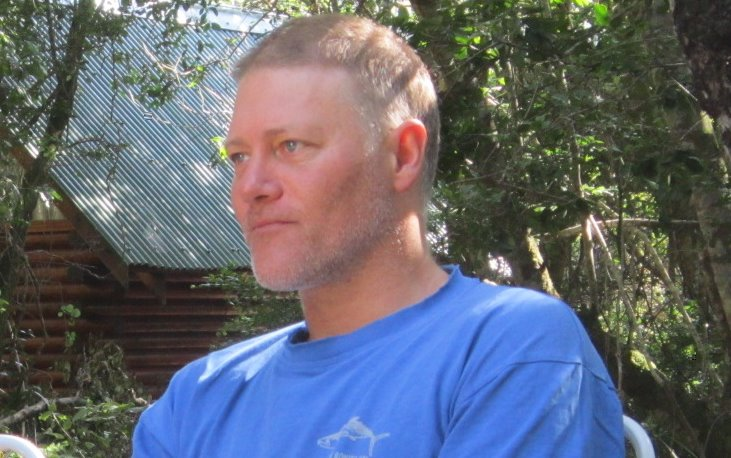
Dr Niko Brümmer
Chief Scientist
AGNITIO, South Africa
The Role of Proper Scoring Rules in Training and Evaluating Probabilistic Speaker and Language Recognizers
It is obvious how to evaluate the goodness of a pattern classifier that outputs hard classification decisions --- you count the errors. But hard classification decisions are implicitly dependent on fixed priors and costs, so that they are applicable only in a narrow range of applications. A classifier can widen its range of applicability by outputting instead soft decisions, in the form of class probabilities or likelihoods. However, it is much less obvious how to evaluate the goodness of such probabilistic outputs. To evaluate the goodness of recognized classes, they can simply be compared to the true class labels in a supervised evaluation database. But we simply don't have a similar truth reference for probabilistic outputs.
A solution to this problem, originally from weather prediction, called "proper scoring rules", has been known for several decades, but has enjoyed only limited attention in pattern recognition and machine learning. This talk will explain how they work, how they generalize error-rate, how they measure information and how to use them for both training and evaluation of probabilistic pattern recognizers.
Short Biography
Niko Brummer received B.Eng (1986), M.Eng (1988) and Ph.D. (2010) degrees, all in electronic engineering, from Stellenbosch University. He worked as researcher at DataFusion (later called Spescom DataVoice) and is currently chief scientist at AGNITIO. Most of his research for the last two decades has been applied to automatic speaker and language recognition and he has been participating in most of the NIST SRE and LRE evaluations in these technologies, from the year 2000 to the present. He has been contributing to the Odyssey Workshop series since 2001 and was organizer of Odyssey 2008 in Stellenbosch. His FoCal Toolkit is widely used for fusion and calibration in speaker and language recognition research.
His research interests include development of new algorithms for speaker and language recognition, as well as evaluation methodologies for these technologies. In both cases, his emphasis is on probabilistic modelling. He has worked with both generative (eigenchannel, JFA, i-vector PLDA) and discriminative (system fusion, discriminative JFA and PLDA) recognizers. In evaluation, his focus is on judging the goodness of classifiers that produce probabilistic outputs in the form of well calibrated class likelihoods.
|
|
|